Description
Automatic optimization has been increasingly used in recent years to investigate turbomachinery while taking into account as many parameters as possible. In this method, the creation (parameterization) and assessment (evaluation) of a machine design is automated. The level of detail of the evaluation can be chosen according to the requirements. The machine design can be evaluated with a simplified design model or by numerical simulations. Whereas numerical flow simulations are very time consuming, replacement models (meta models, surrogate models) are used to reduce the number of necessary simulations without limiting the number of the investigated machine designs that are rendered inadmissible. Replacement models interpolate the operational behavior of previously investigated machine designs to exclude beforehand nonsensical designs during the optimization process. This database is expanded during the optimization process using the current results, thus continually improving the equivalent model.
The automatic assessment of the machine design is based on predetermined optimization criteria’s (e.g. efficiency, surge margin, maximum mechanical stresses). Depending on the selected optimization algorithm and previous results, new promising machine designs are created. The degrees of freedom of the design variations are determined by the parameterization.
As a result of the automatic optimization with multiple optimization parameters, a pareto front is formed. The pareto front is a quantity of machine designs each constitute an optimal compromise between the different optimization criteria.
Relative to the weighting of the optimization criteria, an optimal machine design can be selected.
Field of Application
- Automated improvement of turbomachinery
- Consideration of two optimization goals (also contrary optimization goals)
- Investigation of complex interactions in the machine design
Optimization Methods
- CADO (Tom Verstraete, VKI Brussels) - Metamodell provided differentiell evolutionary algorithm
- Cuckoo Search Algorithmus (Ernst et al.)
- Genetic algorithm (Adamczuk et al.)
Contact
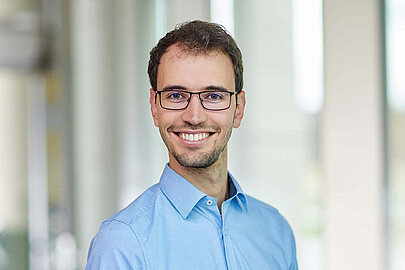
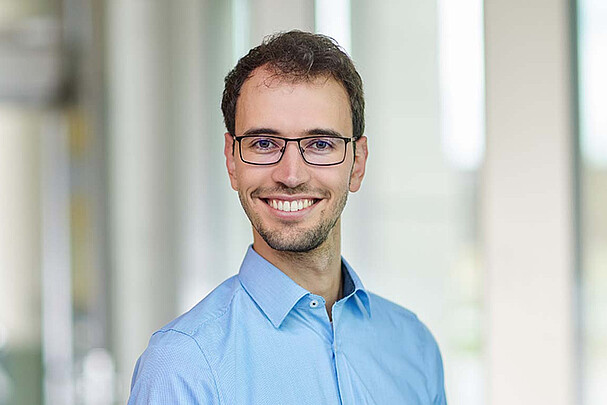
Aeroacoustics, Aeroelasticity and Wind Energy
30823 Garbsen
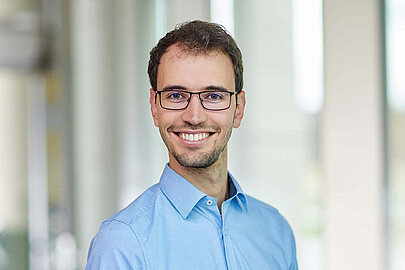
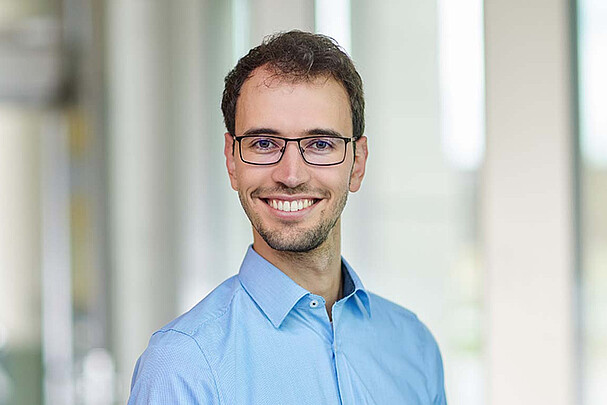
Aeroacoustics, Aeroelasticity and Wind Energy